Enhancing Credit Loss Prediction: The Impact of Regulations and Data Quality in Banks
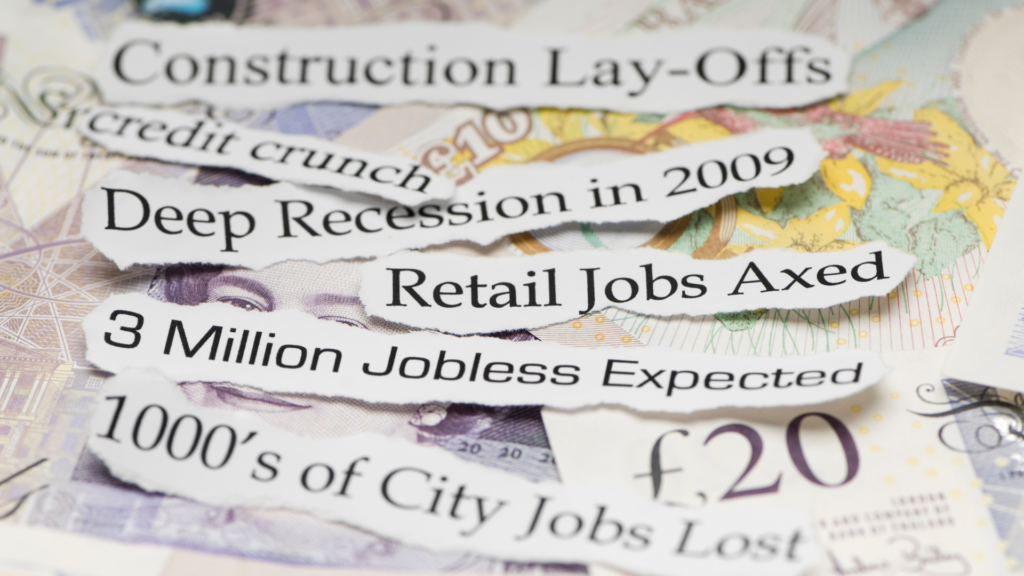

In today’s ever-changing financial landscape, the ability to accurately predict credit loss has become a crucial aspect of risk management for banks and financial institutions. place to enhance the reliability of credit loss forecasts. But to what extent have these rules truly improved the accuracy of such estimates?
Banks now have access to vast amounts of data and sophisticated statistical models to predict credit loss
This includes factors such as historical loan performance, macroeconomic indicators, and borrower-specific information. However, the accuracy of these estimates can still be affected by various factors.
Inaccurate or incomplete data can lead to flawed predictions. Additionally, economic uncertainty can make it challenging to accurately forecast credit losses. Unforeseen events or changes in market conditions can have a significant impact on the creditworthiness of borrowers, making it difficult to predict future defaults.
Regulatory changes and the introduction of new loan products can also affect the accuracy of credit loss estimates
Changes in regulations can require banks to adjust their models and assumptions, which may introduce additional uncertainty. Similarly, the introduction of new loan products with unique characteristics can pose challenges in accurately assessing their credit risk.
Despite these challenges, lenders have made significant progress in improving credit loss forecasting. Estimates tend to be more accurate for similar loan portfolios and during periods of economic stability. This suggests that lenders have been able to refine their models and assumptions to better capture the risks associated with specific loan types.
It is important to note that judgment and bias still play a role in credit loss estimates
While statistical models provide a structured framework for prediction, the interpretation and application of these models require human judgment. Lenders must exercise caution to ensure that their biases do not influence the estimates, as this can lead to inaccurate predictions.
Overall, banks can estimate credit losses reliably but with limitations, particularly during economic turmoil. The rules and regulations implemented in the aftermath of the Great Recession have undoubtedly enhanced the reliability of credit loss forecasts. However, it is essential to recognize that no model or methodology can provide perfect predictions, especially in times of uncertainty.
The benefits of improved credit loss estimation are significant. Accurate forecasts allow banks to allocate appropriate provisions, ensuring they have sufficient capital to absorb potential losses. This promotes financial stability and resilience within the banking system. Additionally, transparent and reliable credit loss estimates reduce the stigma associated with loan losses, fostering trust and confidence in the financial services industry.
Implementing these rules, such as the Current Expected Credit Losses (CECL) and International Financial Reporting Standards (IFRS 9), is not without its challenges. The complexity and cost of implementation can be significant, and there may be increased earnings volatility as a result. However, these rules promote improved risk management practices and transparency, which are crucial for maintaining a stable and trustworthy financial system.
By continuously refining their methodologies and remaining vigilant to biases, lenders can navigate the complexities of credit loss estimation and make informed decisions to protect themselves and their clients.
Interested in speaking with our consultants? Click here to get in touch.