Navigating the Complex Landscape of AI Ethics and Governance
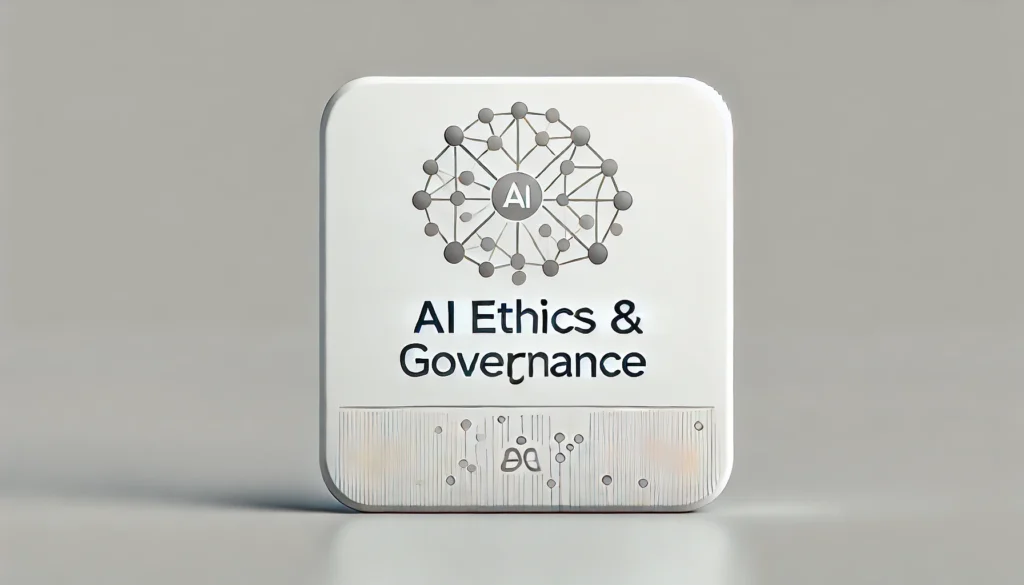

Artificial Intelligence (AI) is revolutionizing industries and reshaping societal norms. However, the rapid adoption of AI technologies comes with significant challenges related to ethics, governance, and societal implications. For organizations leveraging AI, addressing these issues is not just a legal or reputational necessity but also a moral imperative. In this comprehensive article, we explore the critical aspects of AI ethics and governance, examine global regulatory trends, and outline actionable steps for organizations to adopt responsible AI practices.
Understanding AI Ethics: Core Principles
AI ethics is the study and implementation of moral principles that govern the design, development, and deployment of AI technologies. These principles ensure that AI operates in ways that align with societal values and protects individual rights. Below are some of the most critical ethical considerations in AI:
1. Bias and Fairness
AI systems are only as unbiased as the data used to train them. Unfortunately, much of the data available for training AI reflects historical inequities, leading to discriminatory outcomes in applications like hiring, lending, and policing. Addressing these issues requires:
- Dataset Evaluation: Regular audits of training datasets to identify and mitigate biases.
- Algorithmic Fairness Techniques: Implementing algorithms that can adjust for imbalances in data representation.
- Outcome Monitoring: Continuously assessing the fairness of AI outputs across different demographic groups.
2. Transparency and Explainability
AI systems often operate as “black boxes,” making decisions that are difficult for humans to interpret. This lack of transparency undermines trust and accountability. Explainable AI (XAI) aims to demystify these systems, allowing stakeholders to understand:
- The factors influencing AI decisions.
- How data is processed and weighted.
- Potential limitations of the system.
3. Privacy
As AI systems process vast amounts of data, protecting individual privacy has become a paramount concern. Organizations must ensure compliance with data protection laws, such as the General Data Protection Regulation (GDPR), and adopt measures like anonymization and encryption to safeguard sensitive information.
4. Accountability
Determining who is responsible for AI-driven decisions is essential, particularly when these decisions lead to harm or unintended consequences. Accountability frameworks should:
- Clearly define roles and responsibilities within the AI lifecycle.
- Include mechanisms for redress in cases of harm.
- Foster a culture of ethical accountability among developers and operators.
The Importance of AI Governance
AI governance refers to the frameworks, policies, and strategies that ensure the ethical and responsible development and use of AI technologies. Effective governance helps organizations navigate regulatory landscapes, manage risks, and uphold ethical standards. Here are the key elements of AI governance:
1. Regulatory Compliance
Adhering to local and international AI regulations is a foundational aspect of governance. For instance, the European Union’s AI Act requires organizations to classify AI systems based on risk and implement safeguards accordingly. Non-compliance can lead to significant legal and financial penalties.
2. Risk Management
AI systems introduce various risks, including operational failures, ethical lapses, and reputational damage. Organizations must develop robust risk management frameworks that:
- Identify potential risks at each stage of the AI lifecycle.
- Implement mitigation strategies, such as testing and validation protocols.
- Monitor systems continuously to identify emerging risks.
3. Ethical Standards
Ethical standards provide a moral compass for AI development and deployment. These standards should be aligned with organizational values and societal expectations, addressing issues such as inclusivity, fairness, and environmental sustainability.
4. Stakeholder Engagement
Effective governance requires input from a diverse range of stakeholders, including developers, policymakers, end-users, and civil society organizations. Engaging stakeholders ensures that AI systems are designed with a holistic understanding of their societal impact.
Global Perspectives on AI Regulation
AI regulation is a complex and evolving field, with countries and organizations adopting different approaches based on their unique priorities and challenges. Below, we examine key regulatory developments around the world:
1. The European Union
The EU is at the forefront of AI regulation with its proposed AI Act. Key features of the act include:
- Risk Categorization: AI systems are classified as high-risk, limited-risk, or minimal-risk, with corresponding obligations for developers.
- Transparency Requirements: High-risk systems must disclose how decisions are made and provide mechanisms for human oversight.
- Accountability Measures: Organizations must document their AI processes to ensure traceability and compliance.
2. The United States
In the United States, regulatory efforts are more fragmented, with individual states and agencies taking the lead. The National Institute of Standards and Technology (NIST) has developed an AI Risk Management Framework that emphasizes:
- Transparency.
- Fairness.
- Reliability and robustness.
3. The United Nations
The UN has called for global cooperation in regulating AI, emphasizing that AI’s societal impacts require a coordinated international response. The UN’s initiatives focus on:
- Promoting ethical standards.
- Addressing the digital divide.
- Encouraging sustainable AI development.
Implementing Responsible AI Practices
Organizations can take proactive steps to ensure their AI systems are ethical, transparent, and aligned with societal values. Below are practical recommendations for implementing responsible AI practices:
1. Develop Ethical Frameworks
An ethical framework serves as a guideline for decision-making throughout the AI lifecycle. Key components include:
- Mission Statement: A declaration of the organization’s ethical priorities.
- Ethical Principles: A set of values, such as fairness, transparency, and inclusivity.
- Implementation Strategies: Practical steps for embedding ethics into AI design and deployment.
2. Address Bias
Bias in AI systems can lead to unfair outcomes and reputational damage. Strategies for mitigating bias include:
- Diverse Datasets: Collecting data that reflects the diversity of the populations the AI system will serve.
- Algorithmic Adjustments: Using techniques like re-sampling, re-weighting, and adversarial debiasing.
- Independent Audits: Engaging third-party experts to review datasets and algorithms.
3. Foster Transparency
Transparency is critical for building trust and accountability. Organizations should:
- Provide clear documentation of AI systems, including their objectives, limitations, and decision-making processes.
- Offer explanations of AI decisions to stakeholders, particularly in high-stakes applications like healthcare or criminal justice.
- Use tools and techniques that enhance interpretability, such as saliency maps and decision trees.
4. Continuously Monitor and Evaluate
AI systems are dynamic and can evolve in ways that introduce new risks or ethical concerns. Continuous monitoring ensures that systems remain aligned with organizational goals and ethical standards. Key practices include:
- Regular Audits: Periodic evaluations of AI systems for compliance and fairness.
- Feedback Loops: Incorporating user feedback to improve system performance and address concerns.
- Scenario Testing: Simulating real-world scenarios to identify potential vulnerabilities.
The Societal Implications of AI
The integration of AI into society presents both opportunities and challenges. Below, we explore some of the most significant implications:
1. Economic Impact
AI has the potential to drive economic growth by increasing productivity and enabling new business models. However, it also raises concerns about job displacement, particularly in industries susceptible to automation. Addressing these concerns requires investments in workforce reskilling and education.
2. Social Dynamics
AI influences social dynamics in profound ways, from shaping public opinion through targeted advertising to influencing hiring decisions. Ensuring that AI systems are fair and transparent can help mitigate negative social impacts.
3. Environmental Sustainability
Developing and operating AI systems requires significant computational resources, which can have a substantial environmental footprint. Organizations should prioritize energy-efficient AI technologies and consider the lifecycle impact of their systems.
Conclusion
The responsible development and governance of AI are essential for ensuring that these technologies contribute positively to society. By addressing ethical concerns, adopting robust governance frameworks, and staying informed about regulatory developments, organizations can navigate the complexities of AI with confidence.
AI’s transformative potential is immense, but so are its risks. Balancing innovation with responsibility is not just a challenge—it is an opportunity to shape the future of technology in a way that aligns with our highest values. Through continuous learning, collaboration, and a commitment to ethics, we can unlock AI’s potential while safeguarding the principles that underpin our societies.
Interested in speaking with our consultants? Click here to get in touch
Some sections of this article were crafted using AI technology