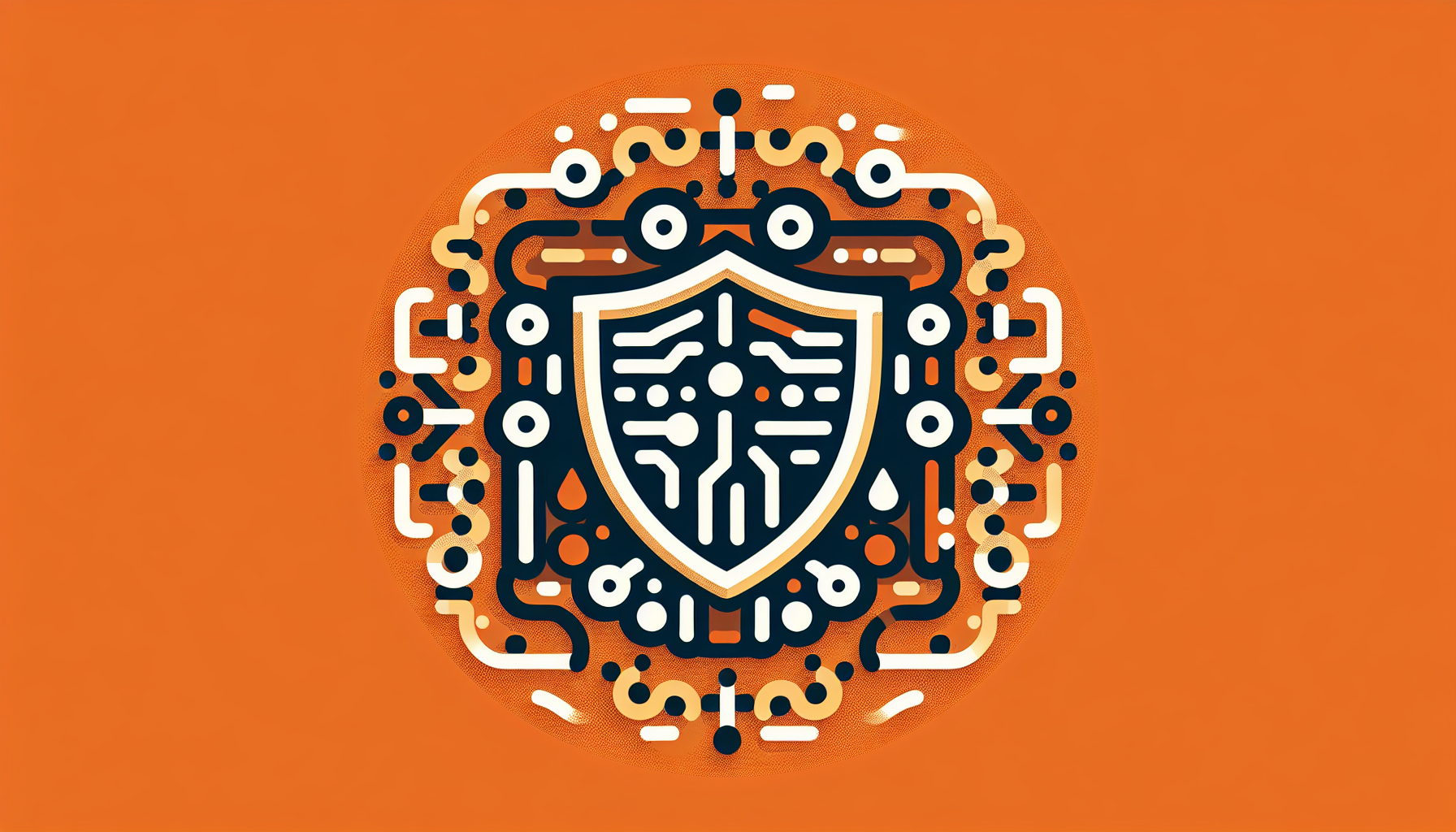
AI Risk Assessment: Identifying and Mitigating Potential Dangers
Introduction
Given the expanding reach of artificial intelligence (AI) across industries and capabilities, the need for AI risk assessment has never been more critical. AI risk assessment is the methodical examination of the potential risks related to AI technologies. It is the fundamental task of recognizing and assessing risks systematically to guarantee the safe and secure operation of AI systems. The significance of risk assessment for AI is evident in the prevention of unintended consequences resulting from AI deployments, such as biased decision-making, data privacy violations, and system malfunctions.
Addressing these risks is fundamental to unlocking the full benefits of AI technology, while mitigating harms. Effective strategies for mitigating AI risk encompass strong security protocols, routine evaluations, and ethical standards tailored for distinct AI uses. By placing a priority on AI risk assessment and mitigation, organizations can cultivate trust, enhance system efficiency, and adapt confidently to the changing AI landscape. Laying a firm groundwork for AI risk management allows organizations to exercise responsible and sustainable innovation.
Understanding AI Risks
The advancement of artificial intelligence (AI) has brought significant improvements to many industries, but it has also introduced a variety of AI risks that must be carefully managed. Understanding these risks is essential for the responsible development and deployment of AI systems.
One major category of AI risks is ethical risks. These risks emerge when AI systems make decisions that could harm individuals or society. For example, algorithms might inadvertently amplify biases in their training data, resulting in unfair outcomes. Promoting fairness, transparency, and accountability in AI decision-making is critical for addressing these ethical issues.
Technical risks are another key area of concern. AI systems rely on massive amounts of data and sophisticated algorithms, which can be a source of errors and unintended consequences. A technical malfunction in an AI-based application, such as autonomous vehicles or medical diagnostics, could have potentially catastrophic consequences. Ensuring the reliability and safety of AI systems requires thorough testing and continuous monitoring to mitigate these risks.
In addition to ethical and technical risks, there are also significant societal risks associated with AI. The widespread adoption of AI technologies may disrupt labor markets, displacing and eliminating jobs. This could lead to economic inequality and social upheaval unless efforts are made to reskill workers and create new employment opportunities. The use of AI for surveillance poses privacy risks and the possibility of abuse by authoritarian regimes, threatening civil liberties.
In conclusion, the ethical, technical, and societal risks of AI must be identified and managed as AI technologies develop. This approach will allow us to leverage the benefits of AI while minimizing its drawbacks, ensuring that AI contributes positively to society in the future.
Through sector-wide adoption of Artificial Intelligence (AI) in today’s fast-evolving societal landscape, alongside the unparalleled opportunities also come conceivable perils. As AI progresses, so too does the critical question of how to uncover such risks to prevent harm and manage risk. Effective identification of these risks, in the context of individual sectors, will be key to maximizing the benefits of AI developments, while securing the operational resilience within those areas.
Methods of Identification
Identifying risks associated with AI demands a varied toolkit. Among the most conventional methods is risk assessment – testing the AI system to expose potential vulnerabilities and anticipated incidents that result in harm. These tests typically screen AI models for signs of data biases, violations of privacy, and ductility defaults. Regular audits to these assessments offer proper coverage of new and unfamiliar threats that were unobserved upon initial deployment.
Scenario analysis is another essential approach for identifying hazards, predicting how the AI may react to unforeseen scenarios in hypothetical judgment situations. It prepares organizations for the dangers and triggers behind the scenes and allows time for the emergency plans to be put in place. In addition, comprehensive knowledge sharing between developers, users, and other experts might reveal a world of risk that AI overlookses.
Sectoral Examples of Risk
Every sector will see its own risks when dealing with the introduction of AI, thus ensuring individual sector-wide analysis remains a priority. Healthcare could, for example, witness the inadvertent issuing of bad advice or advice on treatments following incorrect AI diagnosis. This may be a product of biased or false knowledge leading to wrong decisions on patient care. In this case, it is essential to use methods of identification, such as very rigorously testing the AI against validated clinical trial data sets, to prevent any harm being caused by the AI.
In finance, the problems might unintentionally come from the AI-powered trading algorithms when they act in the conditions of incomplete data or biases in data. This could result in catastrophic financial loss, potentially market manipulation or worse, criminal sanctions against the perpetrators. To counter such risks, financial organizations might use ongoing tracking and stress tests to ensure algorithms operate within their predefined risk standards.
In the world of autonomous cars, the risks are even more visible. If the AI incorrectly interprets the data coming from the sensors, it could lead the car to an accident, putting both the lives of the passengers and the communities in which these systems operate at risk. Testing regimes and real-world pilot schemes can be used to identify the ‘unknown unknowns’ of where a self-driving car might fail.
In sum, identifying risks of AI should be individual sector-wide assessments supported by a variety of methods of identification. These routines will allow our industries to benefit significantly from the potential of AI, while still in control of the risks and ensuring a safe application of innovation in our day-to-day lives. In a world defined by the continuous development of AI, the identification of threats will, therefore, remain crucial.
Mitigation Strategies for AI Risks
With the rapid advancement of artificial intelligence (AI), it is important to develop effective mitigation strategies for handling the risks posed by AI technologies. These strategies are necessary for promoting the ethical progression and application of AI systems, both for the protection of individuals and society as a whole. The main mechanisms to reduce these risks consist of various combinations of policy, regulation, and technical intervention.
Policy and Regulation are central to establishing the basis for mitigating AI risks. Governments and institutions need to create cohesive policies that steer the ethical use of AI, including laws that define standards and requirements for the development of AI in accordance with societal values and human rights. When AI developers and operators are compelled to obey to these laws by regulatory bodies, it helps to maintain transparency and accountability, as well as to secure public trust in AI technologies.
Alongside regulatory mechanisms, it is important to rely on technical means to mitigate the risks associated with AI. Among the technical interventions, one of the most fundamental is the integration of strong security measures to protect AI systems from cyber attacks and misuse. Developers should also focus on developing AI models that are transparent and interpretable, aiding the end user in understanding how decisions are made and addressing any biases or unintended consequences that could be generated by AI algorithms.
Moreover, frequent audits and evaluations of AI systems present a potential technical measure to ensure continuous compliance with safety requirements. Through these assessments, weaknesses and areas of improvement can be identified, thereby guaranteeing that the AI systems work reliably and responsibly under all circumstances.
In order to spur effective action, there is a need for collaboration across domains. Policymakers, private sector leaders, and technical experts should cooperate in establishing guiding policies and sharing best practices, thereby forging a more integrated strategy for mitigating the risks posed by AI.
In summary, the mitigation of AI risks is a multidimensional effort that results from the combination of policy, regulation, and technical effort. Through the implementation of comprehensive policies, the establishment of rigorous regulatory frameworks, and the deployment of sophisticated technical remedies, society will be better positioned to capitalize on the advantages of AI, while keeping the risks associated with AI to a minimum, and thereby advancing to a safer and more ethical technology future.
Enabling Responsible AI Development
In the fast-pace world of technology today, enabling responsible AI development has never been more important. With its growing impacts on society, application, and industries, adhering to the tenants of responsible AI is key to building trust, safety, and effectiveness.
At the foundation of responsible AI are core principles that steer the ethical development and use of AI. These encompass fairness, transparency, accountability, privacy, and security. Fairness guarantees that AI won’t reproduce or even compound biases, promoting equity and inclusivity. Transparency allows for an understanding of AI decision making for stakeholders, which in turn fosters trust. Accountability ensures developers and organizations are held liable for AI outcomes, establishing remedies if mistakes are made and harms are incurred. Privacy upholds the protection of user data by honoring individual rights and liberties, while security defends AI systems against malicious attacks.
Promoting excellence in AI development means to continuously assess and improve. Ethical considerations should be made top priority from inception to deployment. Routine audits and check-ups of AI systems can unveil any biases or vulnerabilities, while interdisciplinary collaboration can introduce diversity of perspectives to AI initiatives. Engaging stakeholders, such as users and policymakers, can boost cohesion of AI with societal values. Awareness and adherence to legal and ethical regulations stipulated by governing bodies are key to following the law and having a valid standing.
By embedding these principles and practices into AI development, we can shape a technology that not only propels progress, but takes into account the welfare of society.
Ultimately, recognizing the significance of assessing AI risk is paramount in our fast-paced digital world of today. Across the conversation, there has been a clear call for a complete and holistic evaluation framework of AI systems. This conclusion solidifies the idea that preventing issues through risk management will protect against backlashes, embedding ethics and reliability into AI application. Reiterating the necessity of these assessments lends a hand to organizations in expecting obstacles, encouraging the conscious use of AI and sustaining confidence from stakeholders. Through continuously perfecting the practice of AI risk assessment, businesses are able to eliminate threats, whilst utilising AI effectively for innovation and prosperity in a safe and ethical manner.