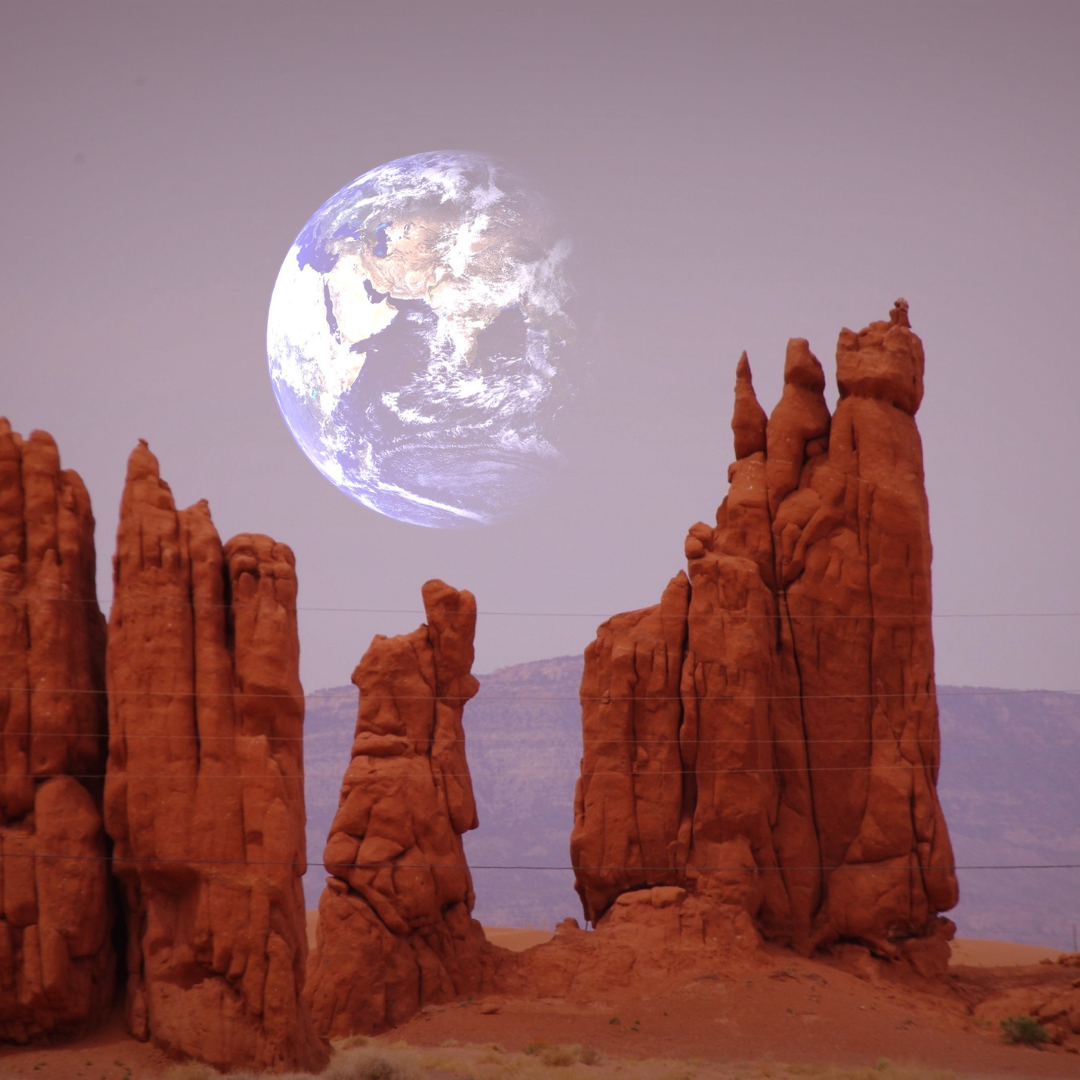
AI Risk Management: How Do You Minimize AI Harms?
In the fast-paced world of technology today, AI risk management has become an essential area of focus. With the increasing incorporation of artificial intelligence in every sphere of life, the management of risks associated with its application is paramount. AI risk management is essentially about the identification, evaluation, and prevention of potential harms from the adoption of AI technologies. While AI represents a significant paradigm shift offering immense opportunities, it also poses potential risks that need to be addressed proactively. The issues of data privacy violation, algorithmic discrimination, and mistakes in autonomous decision-making highlight the importance of minimizing negative impacts of AI. Through effective AI risk management, organizations can prevent undesirable consequences that may undermine the benefits of their AI applications to both society and the economy. As we stride towards an AI-led future, the mitigation of risks will not only protect the interests of stakeholders but will also instil trust and encourage innovation in AI technologies.
Understanding AI Risks
The rapid progress of artificial intelligence (AI) has brought many benefits, but understanding the underlying risks of AI is crucial for both society and businesses. Identifying and managing these risks is key to successfully integrating AI across sectors.
Acknowledged AI risks include algorithmic bias. If AI systems learn from biased data, such systems may unintentionally perpetuate existing prejudices. In turn, this could lead to discrimination in sensitive areas such as hiring, lending, and law enforcement. For example, posing questions with bias within AI may lead to unfair hiring or distorted credit scoring, raising significant ethical concerns.
There is also a risk that AI displaces jobs. A more capable AI system raises fears of replacing human work in certain industries, resulting in fundamental changes to society through shifts in employment and potential increases in the socioeconomic gap if there is no proper training or transition plan in place.
AI can introduce privacy and security vulnerabilities. Systems that process and analyze large amounts of data can attract cyber attacks that compromise sensitive data. Furthermore, a lack of transparency in AI decision-making (or the “black box” output) could undermine trust among the public and business stakeholders.
The consequences for companies may be severe. Financial consequences from customer data breaches or reputational damage from biased AI decisions are risks businesses face. Businesses must also contend with the ever-changing regulatory landscape as governments move to legislate AI governance.
For society at large, the problem is wide-reaching. The ethical dilemmas of AI blur privacy boundaries and prompt the need for novel legal considerations. Collaboration between technologists, ethicists and policymakers is essential to tackle these risks. Businesses might find that managing risks and adopting ethical AI frameworks can help to ensure AI technologies act as a force for good and progress while not compromising human values.
Techniques of Risk Management: Stepping with Certainty through Uncertainties
Risk management is a critical domain for companies that intend to tread the fine line between stability and progression. With a sound risk management framework in place, firms can skillfully steer through uncertainties and protect their vested interests. This article discusses different modalities of risk management and underscores the importance of proactiveness, featuring futuristic technological precisions including AI, in mitigating potential perils.
Migrating into Variegated Risk-Management Strategies
- Risk Identification and Evaluation:
The keystone of a sound risk management strategy is the identification of risks and an assessment of its repercussions. The firm ought to conduct a comprehensive risk analysis to blemish out risk-prone areas and set the stage for tailored interventions. - Risk Curtailment via Diversification:
Diversification is an effective stratagem wherein, one spreads out investments and operations across diversified activities or geographical locations, thereby diluting the impact of localized risks and championing a more robust business composition. - Frequent Monitoring and Appraisal:
A crucial yet glossed-over facet of risk management is a perpetual monitoring. Periodical reappraisal of risk profiles empowers businesses to adapt speedily to changing dynamics, ensuring that potential risks are addressed prior to them burgeoning into weighty predicaments. - Preparation with Contingency Planning:
A well-knitted contingency planning serves as a potent safety catch. It encompasses delineating well-laid reactions for potential risk situations, and indulges a structured reaction blueprint to unforeseen circumstances, thus reducing downtime and financial harm. - Leveraging Insurance:
Insurance retains a nucleus amid the geo-political uncertainties, proffering financial hedge against specific risks. By estimating and procuring apt insurance covers, companies can insulate themselves from unanticipated financial liabilities.
Underscoring the Relevance of Proactive Strides
The proactive strides stand as a cornerstone to efficacious risk management conservative against the obsolete reactive stances, warranting the voguish responsive and anticipatory approach of the present. Enterprises practicing proactive strides can foresee and resolve potential impediments afore they morph into setbacks.
Assimilating AI and Advanced Analytics:
The convergence of AI in risk management strategies has been an epoch-making venture. AI devices can prefigure potential risks by combing through colossal data reservoirs and deciphering unspoken patterns which human analyzers can oversee, permitting entities to address qualms with exactitude and timeliness.
- Installing Strong Training Regimens:
Fueling the staff with an understanding and acumen for identifying and riposting threats engenders a proactive tenor. Periodic grooming sessions ascertain readiness of the workforce in stride with the company’s posture on risk management. - Embarking on Industry Benchmarking:
Staying abreast of industry norms and undertakings unearths victorious strategies of risk mitigation espoused by the fellows, facilitating proactive course modifications and emboldening risk management norms.
In culmination, nurturing a culture of proactive risk management enfolding a spectrum of strategies ranging from encompassing review to Augmented AI prognostications can substantially shore up a firm’s resilience. In consequence, firms not just shield its estates but steers way for sustainable progression during uncertainties.
Case Studies of Successful Management of AI Risks
The adoption of Artificial Intelligence (AI) in business processes has led to leaps and bounds in technological progression. But with progression, comes risk. Understanding how companies manage these risks is critical. Observation of successful AI risk management through case studies provides critical insights and best practices for businesses in need of safe AI deployments.
Notable in this field is the work of IBM — a pioneer in AI technology. IBM has implemented a complete strategy for managing AI risks including ongoing monitoring and validation of AI systems. IBM’s use of an AI Ethics Board ensures ethics are at the core of their AI innovations. This highlights the importance of creating governance structures that supervise AI operations, a best practice other organizations can mimic to increase transparency and accountability.
Also in technology, Google has created powerful devices like the “What-If Tool” to facilitate responsible AI experimentation. The tool lets developers visualize machine learning models and identify potential biases — a key aspect of risk management. Google’s case shows necessity to arm AI teams with tools that boost deep understanding of AI behavior and potential challenges. The active identification and neutralization of AI biases is a major method to successfully managing AI risks.
JPMorgan Chase, a financial services firm, has engineered advanced AI risk strategies with a focus on fraud prevention and customer support. This involves using machine learning models in strict observance of regulatory compliance to decrease the risk of financial loss. The takeaway from this study is the importance of harmonizing AI strategies with regulatory conditions to eliminate risks.
Best practices in handling AI risks gleaned from these case studies dictate the need for dedicated ethical review and governance. Secondly, investment in resources that offer insight into AI processes is vital for identifying and managing ingrained risks. Lastly, all AI strategies, particularly in sectors with high regulatory needs, must comply with prevailing regulatory norms.
These practical cases prove that successful AI risk management goes beyond standardized solutions. Instead, it requires personalized solutions that harmonize with specific business contexts, ensuring ethical usage of AI technology.
Future of AI Risk Management: Navigating Emerging Trends and Challenges
Looking ahead, AI risk management in the future will chart new territories of technical surveillance as AI technology progresses. With the fast pace of innovation, various future trends will significantly change how organizations approach this critical domain.
A primary trend is the use of AI systems in making decisions in all industries, requiring a solid approach to deal with potential AI-related risks, such as bias, privacy issues, systemic failures, etc. Handling these issues proactively to make AI fair and accountable will be key to overcoming them.
Additionally, with the expansion of AI in areas like finance and healthcare, new forms of risk come into play. Increasingly dealing with sensitive and mission-critical data through AI needs robust criteria for evaluation; continuous monitoring and testing to assess its compliance with regulations and ethical requirements. Hence, evaluating the impact of AI is essential to guaranteeing public trust and security.
To tackle future risks in AI successfully, companies need to promote continuous review and adaptation to new technologies. This way their AI systems remain up-to-date with current standards and correlated emerging threats. Adopting adaptive risk management will help in handling unexpected challenges and keeping up with the pace of technological change.
In sum, the future of AI risk management involves identification of upcoming trends, adjusting to the challenges they present and maintaining a rigorous process of evaluation. By being proactive and developing adaptive methodologies, firms can secure their AI systems and stakeholders efficiently.
The key message for businesses is to develop and implement clear policies and procedures not only to ensure compliance, but to build stakeholder trust. In other words, well-designed governance underpins business value deployment of AI.
Leave a Reply