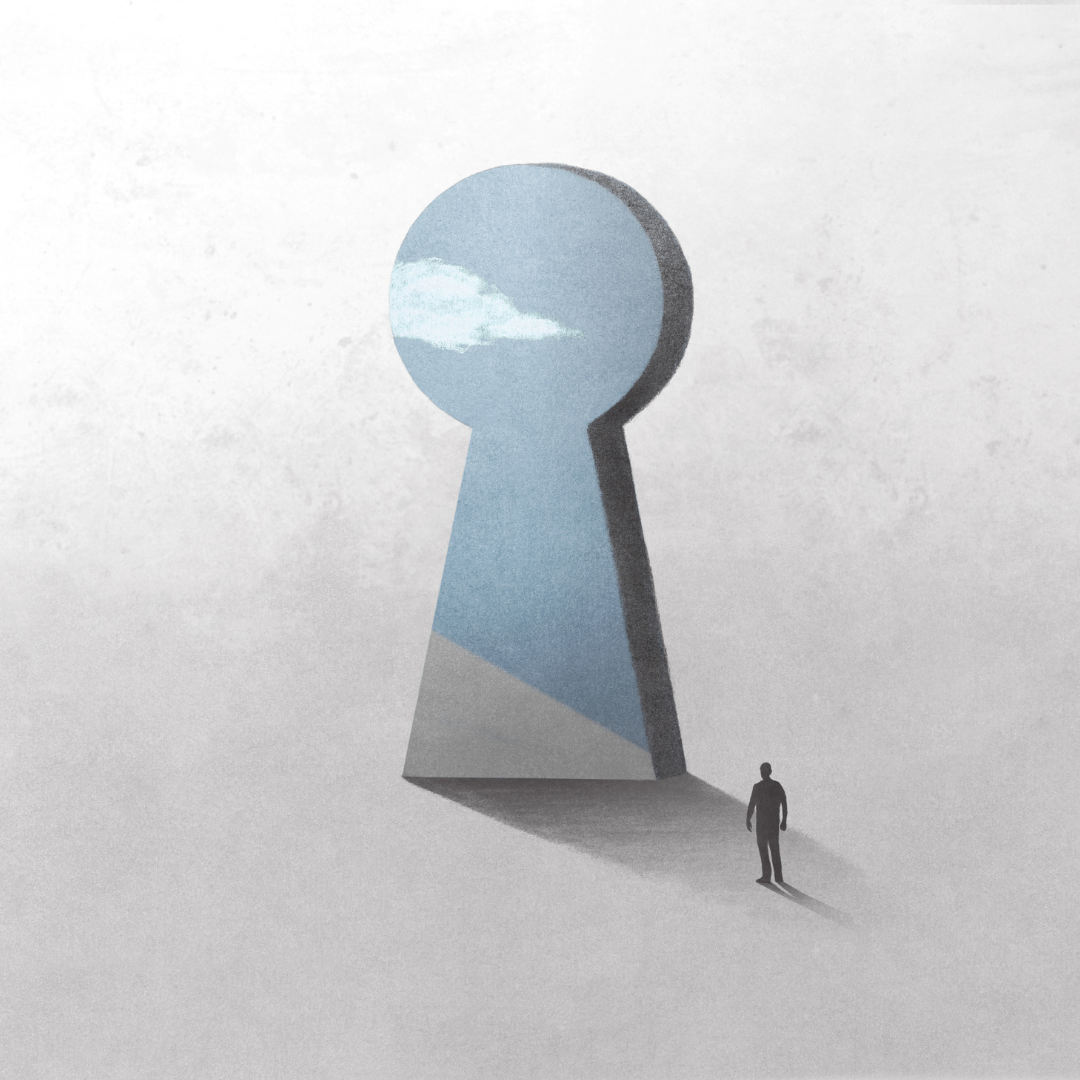
AI Risk Appetite: What Factors Determine Your Ideal Level?
In the fast-paced digital environment of today, the notion of AI risk appetite has gained importance as a key consideration for businesses and developers. AI risk appetite refers to the amount of risk that an organization is willing to accept when adopting Artificial Intelligence (AI) technologies in its operations. Identifying risk in adopting AI is critical in making strategic choices about how and why to use it, ensuring that the deployment is in line with an organization’s goals and compliance mandates.
Knowing its risk appetite allows organizations to leverage the transformative capabilities of AI while managing any potential downsides. This includes evaluating considerations such as data privacy, algorithmic bias and job displacement. By having a clear view on risk, businesses can achieve a balance between innovation and accountability, providing a solid basis for the sustainable integration of AI. Such forward-thinking steps not only protect the company but also build trust among stakeholders, opening the path to innovation in the AI age.
What is AI Risk Appetite?
In the ever-changing landscape of artificial intelligence, having an understanding of and defining “AI risk appetite” is vital for organizations that are leveraging AI. With a comprehensive understanding of AI risk appetite, companies can link AI-driven initiatives to their broader safety and strategic goals, mixing innovation and risk at an acceptable level.
Definition of AI Risk Appetite
AI risk appetite is the amount and type of risk that an organization is prepared to take in order to meet its AI-related objectives. It encapsulates the limits established by a business for the uncertainty surrounding the use of AI systems. It includes, but is not limited to, how much uncertainty a business is willing to tolerate, ethical concerns, and the effect on stakeholders, all measured rigorously against the potential rewards of the implementation. This strategic oversight guarantees that an artificial intelligence deployment is as much ground-breaking as it is safe and ethically run, cultivating trust and dependability.
Risk Types within AI Systems
To define AI risk appetite, an understanding of the differing risks that are associated with AI systems is essential. Regulatory and compliance risks loom large in an environment where laws concerning the use of AI are always in flux. Ethical risks, particularly about biases in AI models that can lead to prejudice consequences, also feature. Operational risks, the possibility of hitches or failures within AI technology that could cause disruption or financial loss, are a consideration. Security risks, some of the more pertinent ones, concern breaches in data privacy and the chance of AI systems being targeted in an immoral fashion.
Through the categorization of these risks, the level of potential impact and probability can be better understood. A robust AI risk management strategy requires not just the identification of these risks but the outlining of responses which contain their impact while aligning with the organization’s AI risk appetite.
In short, by defining AI risk appetite, an organization is laying the cornerstone for its conscientious deployment of AI technologies, making sure they are doing this in a way that sits within their own equilibrium of innovation and risk aversion.
Factors Influencing AI Risk Appetite
When organizations decide to employ artificial intelligence, one of the key considerations is their AI risk appetite. Such appetite is shaped by a range of factors that determine the level of risk an organization is willing or able to accept in the context of AI ventures. An understanding of these factors can assist companies in making informed choices about the integration of artificial intelligence into their operations.
Internal Factors
Internal considerations are pivotal to the determination of an organization’s AI risk appetite. Chief among internal considerations is the goals and objectives of the organization itself. A company with ambitious growth targets might take on more risk and adopt innovative AI technologies despite uncertainties, whereas one focused on stability and long-term viability could be more conservative, emphasizing the need for due diligence and a pilot study before full-scale implementation.
Another crucial internal motivation is the availability of resources — financial, technical, or human. An organization with ample resources might be more able to afford to gamble on high-risk AI initiatives, with resource-constrained organizations focusing on less risky, more incremental AI adoption. Furthermore, the organization’s internal culture and risk policy — including how well prepared the organization is to manage setbacks — can strongly influence its AI risk appetite. An organization with a robust risk management structure and a culture that supports innovation would likely be more willing to take on calculated risks.
External Factors
External determinants are also pivotal to AI risk appetite. Regulation is among the most powerful external influences. Government and sector regulations differ widely and can prescribe the extent to which AI can be employed and the standards to which it must conform. Companies in heavily regulated industries might exhibit less of a risk appetite because of potential consequences from breaching rules, while companies in less stringently regulated sectors might be afforded more freedom and thus be more inclined to experiment with advanced AI solutions.
Market conditions are another crucial external determinant. In markets that are fast-paced and undergoing rapid change, organizations often have a strong need to adopt AI urgently in order to compete, simultaneously accepting more risk. In stable or contracting markets, businesses might prefer to be more conservative, taking a cautious approach to the viability and risks of new AI technologies.
In summary, the interaction between internal and external determinants can greatly help organizations in framing their appetite for AI risk. By aligning the acceptance of risk with their vision, available resources, regulation and market state, organizations can tap into the power of AI while managing the risks involved. This alignment not only fosters a sustainable adoption of AI, but also ensures the continued agility and competitiveness of companies within the ever-changing technology landscape.
Evaluating Your Organization’s AI Risk: A Methodical Approach
Conducting an AI risk assessment for your organization is essential to aligning AI projects with your organization’s strategic goals and ethical standards. Proper risk management could protect your organization from risks and help maximize the value of AI. A structured approach to assessing risk, complete with assessment tools, can provide a thorough evaluation.
Step 1: Identify AI Applications and Stakeholders
Begin by creating an inventory of all AI applications within the organization. This includes which departments use AI and the stakeholders working on AI initiatives. Understanding the scope and people involved with AI lays the foundation for a detailed risk assessment.
Step 2: Define Risk Categories
Different AI applications bring different risks. Define risk categories that are relevant to the operations of your organization, for example, data privacy, algorithmic bias, regulatory compliance, and business continuity. Categorizing risks helps guide the assessment process.
Step 3: Deploy a Risk Assessment Framework
Use a structured framework to assess risk, such as AI Risk Management Framework (AI RMF) or ISO/IEC 27005 for general risk assessments. These frameworks provide a way to identify, analyze, and mitigate the risks associated with AI.
Step 4: Leverage Assessment Tools
Use specific assessment tools and techniques in conducting the risk assessment. Tools such as Google’s What-If Tool and IBM’s AI Fairness 360 can assist in testing bias and fairness in algorithms. Microsoft’s InterpretML is a platform that can be used to explain AI models to manage risk.
Step 5: Perform a Thorough Evaluation
Conduct a comprehensive evaluation using the frameworks and tools. Assess the data vulnerability, algorithmic bias, compliance with laws and regulations, and impact on stakeholders. Employ both qualitative and quantitative methods when assessing the risk.
Step 6: Formulate Mitigating Strategies
After identifying and analyzing the risks, determine the appropriate mitigating actions. This could involve refining data inputs, enhancing algorithm efficiency, enforcing stricter data governance controls, or other measures.
Step 7: Monitor & Adapt Continuously
AI risks change as technology and regulations change. Continuously monitor and update your framework and strategies to have an effective AI risk management program in place.
By using these steps along with applicable assessment tools, your organization can assess AI risk and develop a strategy for proactive risk mitigation. This will ensure that AI projects benefit the business and reduce the potential for harm.
Managing AI Risk
As reliance on artificial intelligence continues to grow, managing AI risk in organizations becomes a critical task. Comprehensive risk mitigations strategies are essential to managing potential threats. One method is to conduct regular AI audits to identify vulnerabilities and ensure compliance with regulations. Another is to establish a clear governance framework that defines roles and responsibilities to promote accountability at all levels. Deploying risk management strategies not only addresses risks but also builds confidence in AI systems.
Exploring documented examples of successful risk management can provide organization with useful lessons for improving their own deployment of AI. For example, a major financial institution was able to reduce AI risk by incorporating bias detection algorithms that promoted fair outcomes in loan decisions, thus preempting bias and safeguarding the company’s reputation. Similarly, a healthcare firm deployed an AI diagnostic tool with a live monitoring system for patient treatment, allowing rapid identification and correction of errors that was critical to safety and reliability of the service offered. These cases highlight the importance of continuous monitoring and adjustment of AI as risks evolve.
In summary, managing AI risk is a multifaceted problem that requires careful, strategic thinking. With risk mitigation methods and insight from successful examples, organizations can deal with the complex challenges posed by AI while ensuring operations and trust. By prioritizing these approaches, organizations can safely exploit AI’s opportunities with less risk.
In summary, managing the nuances of AI risk appetite comes down to thoughtful consideration and thoughtful planning. Companies need to distill key learnings about risk assessment to customize their risk approach. Reflecting on their current risk position and the implications of AI solutions will enable organizations to act in accordance with their strategic objectives. The challenge for organizations is to proactively assess and (if necessary) modify their risk appetite to maintain a flexible and adaptive posture in an era of ongoing technological advancement. Through developing an agile way of dealing with risk, businesses can manage their exposure to risk, while benefiting from opportunities related to AI to drive growth and innovation.
Leave a Reply