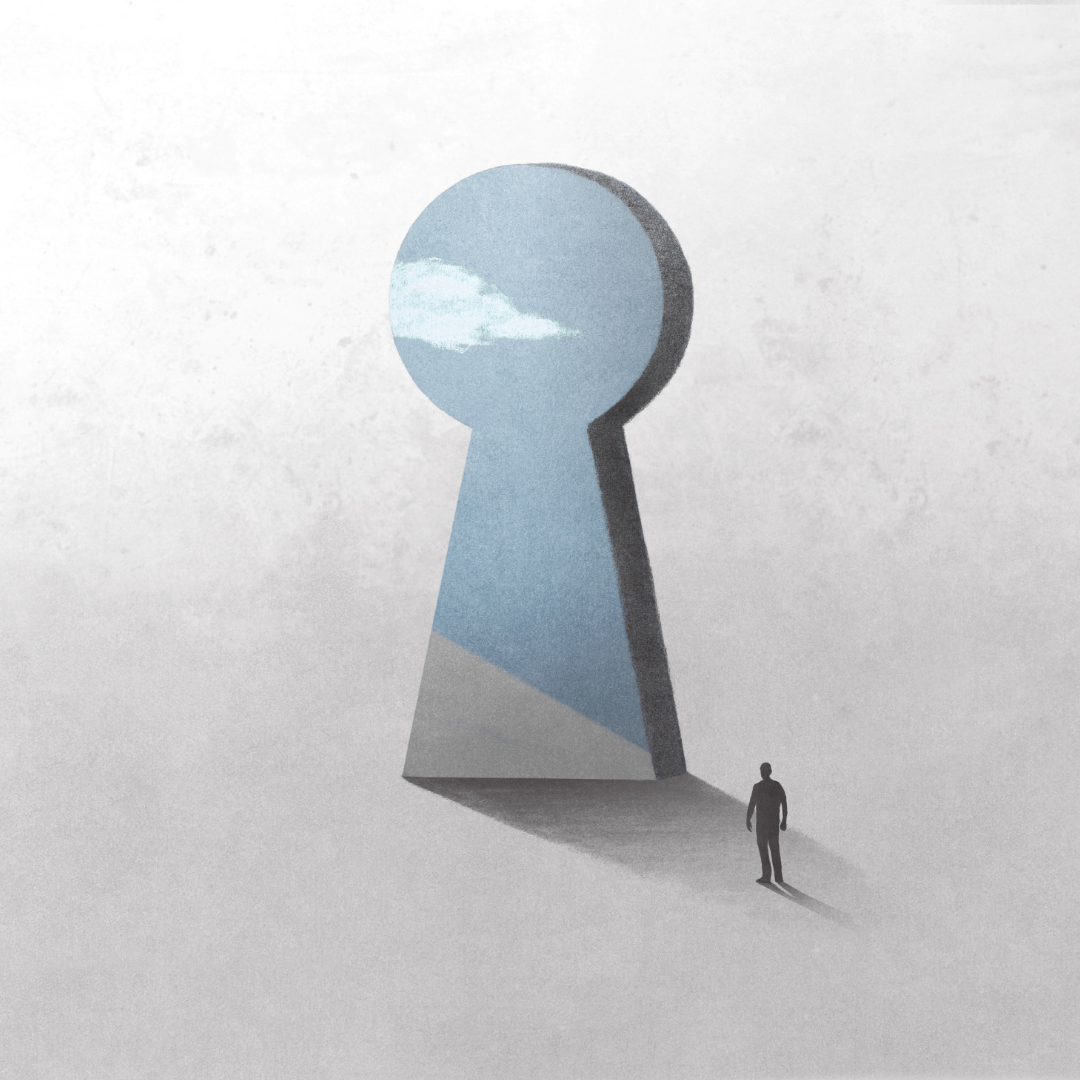
AI Risk Scoring: How to Quantify and Mitigate AI Vulnerabilities
In today’s fast-changing digital world, having an understanding of AI risk scoring is key for both companies and individuals. AI risk scoring is the process of assigning a rating to the possible risks involved in deploying and operating artificial intelligence systems. It is a necessary step to ensure the safe and effective use of AI technology. As AI risks, such as bias, security gaps, and ethical concerns, have become more apparent, there is a need to quantify these problems. By accurately evaluating these risks, companies can differentiate well-informed choices around the use and management of AI. AI risk scoring allows companies to proactively identify threats and thus protect their businesses and gain consumers’ trust. Additionally, by focusing on high-risk areas, businesses can distribute their resources more effectively, targeting issues that could have the most impact. In the world of today’s data and tech, mastering AI risk scoring is key to unlocking the potential of AI, all while controlling the risks that come with it.
Key Takeaways
- AI risk scoring is the process of evaluating and managing the risk of potential problems with artificial intelligence.
- One method of AI risk scoring is quantitative risk assessment, in which mathematical models are used to estimate how likely and severe various AI problems are. This allows risks to be ranked by numeric score to determine what should be focused on.
- Another method is qualitative risk assessment, which involves expert judgment and scenario analysis to identify and understand problems. While subjective, qualitative risks ensure that stakeholders can provide input and that complicated scenarios too complex for numeric methods are considered.
Hybrid methods, such as the combination of quantitative and qualitative methods, are becoming more popular in AI risk scoring. By blending expert opinion with data analysis, such methods provide a more complete view of risk. This blending ensures that both easily scored risks and those with nuance and context are accounted for.
- AI risk scoring is increasingly important as AI systems play bigger roles in decision-making across a variety of domains. Understanding the risks helps in fields like financial services, health care, and autonomous systems, where AI problems could be disastrous. Using advanced methods can help businesses avoid harmful AI mistakes and take full advantage of AI advances in a secure and ethical way.
Understanding the Risks of Common AI Vulnerabilities and Their Implications for Businesses
With advancements in technology, the risks surrounding AI vulnerabilities have become a major issue for companies utilizing AI systems. It is critical to recognize these common vulnerabilities in order to protect business processes and preserve trust with stakeholders.
Data Privacy Violations: One of the common AI vulnerabilities is the threat of data privacy breaches. AI systems often handle large amounts of sensitive data, and vulnerabilities in data processing may result in unauthorized access or data breaches. As a result, companies may face legal consequences and reputational damage.
Bias and Discrimination: AI systems are also at risk of algorithmic bias, which may occur due to biased data or flawed methods of model training. Algorithmic bias can lead to unfair treatment of individuals and biased decision-making, potentially causing customer boycotts and regulatory penalties for companies.
Model Inference Attacks: Companies should be concerned about model inference attacks, in which adversaries use AI models to extract sensitive information. Such attacks may lead to data exposure or loss of a competitive edge brought about by innovative AI efforts.
Impact on Business Operations: The impact of these AI vulnerabilities on a company’s activities can be extensive. Companies may suffer financial damage, a loss of consumer confidence, or operational disruption. There are also enduring consequences, including compliance risks and potential legal disputes that draw resources and focus away from core operations.
Recognizing and remediating these common AI vulnerabilities allows companies to manage risks and operate their AI solutions in a more secure manner, thereby securing continued growth and resilience in an AI-driven world.
How to measure AI risks: a step-by-step guide
As the development of artificial intelligence (AI) technologies progresses, organizations are increasingly recognizing the importance of understanding and mitigating risks associated with AI deployment. Quantifying AI risks is a key element in the responsible and successful implementation of AI. Here is a systematic guide on how to quantify these risks, incorporating necessary tools and frameworks:
Step 1: Identify Risks
The initial step in quantifying AI risks is a thorough identification of potential hazards resulting from the use of AI. This includes the insight into the AI system itself, its environment, and its interaction with users or other systems. The main areas of focus are data privacy, algorithmic bias, system failure, and business disruption. Involving a variety of stakeholders can provide multiple viewpoints and may highlight risks that would be overlooked.
Step 2: Evaluate Severity and Likelihood
After determining potential risks, the next phase is to evaluate the severity and likelihood of the identified risks. This refers to estimating the impact of each risk on the organization and its stakeholders, and assessing the probability of the risk occurring. Data and historical comparisons may be employed in the evaluation, assisting in terms of a structured approach to risk evaluation.
Step 3: Employ Tools and Frameworks
Applying specialized tools and frameworks is imperative for the effective quantification of AI risks. Tools like Fairness Indicators and IBM’s AI Fairness 360 can support in assessing for biases and AI model fairness. Also, frameworks like the NIST-developed AI Risk Management Framework (in the process of development) deliver structured methodologies for the holistic management of AI risks.
By using these tools, organizations are guided not only through the identification of risks, but also on the measurement and handling of these risks to ensure the alignment of AI systems with ethical norms and organizational objectives.
Step 4: Define Mitigation Strategy
In the wake of quantified risks, the formulation of robust mitigation strategies is vital. This might involve the development of AI systems that include contingency actions, the utilization of algorithms that continuously check for biases or anomalies, and the initiation of regular audits and updates. To effectively minimize the impact of such risks, such strategies should be destinationted to the individual risks that were identified in earlier steps.
Step 5: Perpetual Monitor and Review
Quantifying AI risk is not a one-off event, instead, it is an ongoing process. Constant surveillance and periodic revision are essential steps in reaction to the emergence of new hazards with advancements in AI technologies and their specific applications. Feedback loops and adoption of agile models can heighten responsiveness to new dangers for an organization.
In sum, the quantification of AI risks is a complex process demanding a methodical strategy. Through structured steps and employing specialized tools and frameworks, organizations will be better placed to handle the potential hazards linked to AI, ensuring secure and trustworthy AI systems.
Strategies to Mitigate AI Vulnerabilities
As AI becomes prevalent across industries in this ever-changing technological landscape, the security of these systems is critical and presents unique challenges. Efficiently mitigating AI vulnerabilities requires a comprehensive strategy comprising prevention mechanisms and security best practices to protect both data and system integrity.
The first strategy to mitigate AI vulnerabilities involves the enforcement of strong access controls. Limiting system access to authorized personnel only minimizes the exposure of sensitive data and reduces the chance of unauthorized alterations. Moreover, deploying multi-factor authentication and enforcing frequent updates to credential strategies offers additional layers of security needed to safeguard AI frameworks.
The next key strategy is to ensure data integrity by employing rigorous data validation mechanisms. Using high-quality, verified datasets to train AI is fundamental. Confirming data precision helps prevent incorrect decisions or bias that may lead to vulnerabilities. Regularly auditing and monitoring AI systems to detect anomalies early are equally essential for the good health of the system.
Preventative measures are critical components in mitigating vulnerabilities. Regular patch updates are essential. By continually updating software and hardware, organizations can address potential security vulnerabilities before they are exploited. Using encryption technologies to protect data in motion and at rest further prevents unauthorized access and data breaches.
Adhering to AI ethics best practices is another key aspect. Building transparent AI systems with interpretable models empowers stakeholders to comprehend, trust, and monitor AI decisions. This transparency aids in detecting unusual behaviors or biases and ensures adherence to regulations.
Lastly, conducting frequent security training and awareness campaigns for all staff will promote a culture of security hygiene and make organizations more resilient to AI vulnerabilities. Employing such strategies and practices arms companies with the right tools to detect and prevent AI vulnerabilities while preserving the integrity and reliability of AI systems in the digital era.
In summary, the significance of AI risk scoring deployment cannot be overstated within our constantly changing technology climate. By recognizing the value of AI risk scoring, businesses can better identify risks and remediate vulnerabilities. Comprehensive AI risk scoring approaches offer organizations improved decision-making capabilities that preserve safety and regulatory adherence, and also support innovation. Driving adoption of these approaches not only strengthens security practices; it cements a competitive advantage. Treating AI risk scoring as an inherent part of risk management, therefore, defends critical assets and drives continued success, exemplifying its fundamental place in today’s business landscape.