Enhancing Trustworthy AI: Strategies for Banks, Financial Institutions, and Big Tech
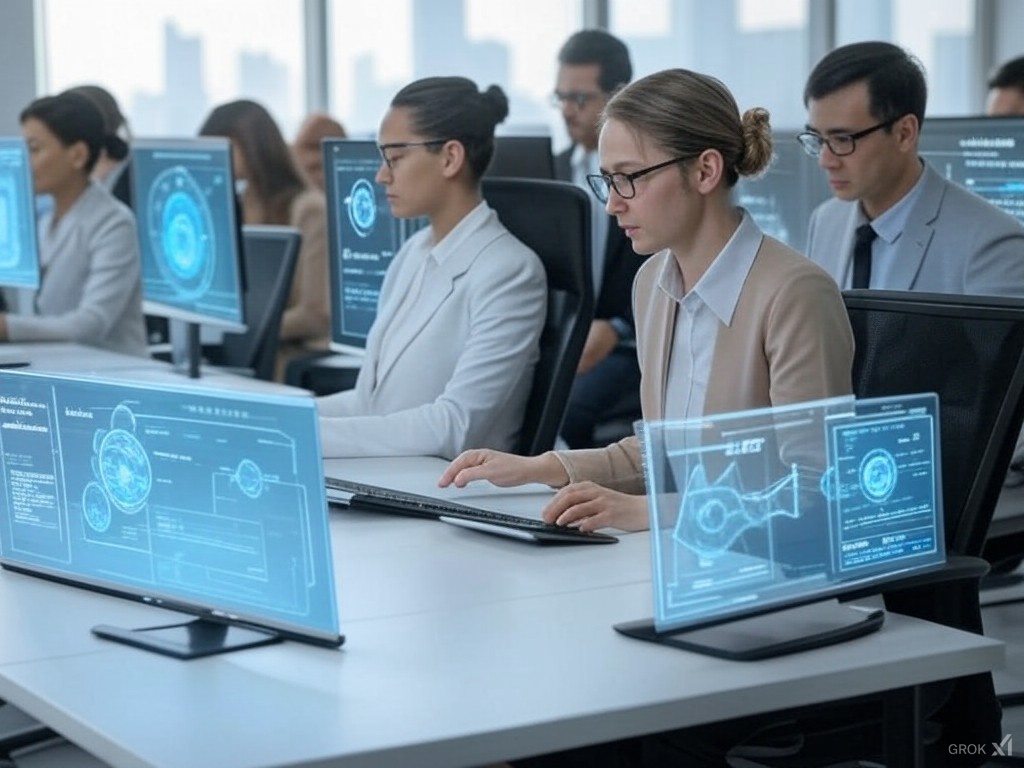
In an era where Artificial Intelligence (AI) increasingly powers critical operations across industries, the trustworthiness of these systems is paramount. For banks, financial institutions, and big tech companies, ensuring AI systems are reliable, ethical, and secure is not only a competitive advantage but also a regulatory and reputational necessity. Drawing from the insights of frameworks like the National Institute of Standards and Technology (NIST) AI Risk Management Framework (AI RMF), organizations can establish robust strategies for AI risk management and trustworthiness. This article explores actionable steps tailored to the unique needs of these sectors.
The Importance of Trustworthy AI in Financial Services and Big Tech
AI has transformed industries by automating processes, enhancing decision-making, and delivering personalized experiences. However, the stakes are especially high for financial institutions and technology giants, where AI systems manage sensitive data, drive critical decisions, and directly impact customer trust. Missteps in AI implementation—such as bias, lack of transparency, or security breaches—can lead to significant financial losses, regulatory penalties, and reputational harm.
Trustworthy AI is not merely about mitigating risks but also about ensuring long-term sustainability and growth. By aligning AI systems with ethical standards, organizations can build stronger relationships with customers, comply with emerging regulations, and maintain a leadership position in the rapidly evolving digital landscape.
NIST AI RMF: A Blueprint for Managing AI Risks
Characteristics of Responsible AI Systems
The NIST AI Risk Management Framework provides a structured approach for organizations to manage AI risks effectively. Its core characteristics emphasize the responsible use of AI systems, aiming to create technologies that are:
- Valid and Reliable: AI systems should perform consistently under expected conditions and adapt to new inputs without compromising their core functionalities.
- Accountable and Transparent: Organizations must maintain clear governance structures and document processes to ensure accountability.
- Safe, Secure, and Resilient: Security is critical to prevent unauthorized access or disruptions.
- Explainable and Interpretable: Stakeholders, including regulators and users, should be able to understand AI decision-making processes.
- Privacy-Enhanced and Fair: AI systems must respect privacy laws and ensure fairness, minimizing biases and disparities in outcomes.
These characteristics serve as a flexible foundation for organizations to align their practices with industry-specific norms, regulations, and ethical considerations.
Applying NIST AI RMF to Financial Institutions and Big Tech
Establishing a Baseline
- Assessment of Current Capabilities: Conduct a thorough evaluation of existing AI systems, identifying areas of strength and potential vulnerabilities.
- Alignment with Enterprise Risk Management (ERM): Integrate AI risk management into broader ERM frameworks, ensuring cohesive governance across the organization.
Risk Measurement and Tolerance
- Develop metrics to assess the performance and reliability of AI systems, focusing on their ability to mitigate risks in real-time.
- Define clear thresholds for acceptable risk, considering the unique operational and regulatory contexts of the financial and tech sectors.
Iterative Risk Management
- Continuously revisit the AI RMF as systems evolve and new technologies are adopted.
- Incorporate feedback loops to refine AI models, ensuring they remain aligned with organizational goals and compliance requirements.
Lessons from Trustworthy AI Frameworks
Diverse frameworks have emerged to address AI risks, including Deloitte’s Trustworthy AI Framework™, which emphasizes six key characteristics:
- Fair and Impartial: Ensure AI systems do not perpetuate discrimination or inequality.
- Robust and Reliable: Build systems that perform as expected under varying conditions.
- Privacy: Protect user data with advanced encryption and secure storage practices.
- Safe and Secure: Safeguard systems against cyber threats and unauthorized access.
- Responsible and Accountable: Assign clear accountability for AI decisions and outcomes.
- Transparent and Explainable: Promote understanding and trust among stakeholders.
By integrating these principles, organizations can strengthen their AI systems’ resilience and trustworthiness.
Key Challenges and Solutions
Addressing Bias in AI Systems
- Challenge: Bias can stem from skewed training data or algorithmic flaws, leading to discriminatory outcomes.
- Solution: Implement rigorous testing and validation processes to identify and mitigate biases. Regular audits and diverse data sources are critical.
Ensuring Explainability
- Challenge: Complex AI models, such as neural networks, can be difficult for stakeholders to understand.
- Solution: Use interpretable models where possible and develop tools to explain decisions made by complex systems in non-technical terms.
Strengthening Security
- Challenge: AI systems are vulnerable to attacks such as data poisoning or adversarial inputs.
- Solution: Adopt robust cybersecurity measures, including encryption, monitoring, and response protocols.
Regulatory Compliance
- Challenge: Rapidly evolving AI regulations can be challenging to navigate, especially for global organizations.
- Solution: Maintain a dedicated compliance team to monitor regulatory changes and ensure alignment with local and international laws.
Steps Toward Building Trustworthy AI
Define Clear Objectives
Establish clear goals for AI implementations, focusing on outcomes that align with organizational priorities and ethical considerations.
Enhance Collaboration
Foster collaboration between data scientists, ethicists, legal experts, and industry regulators to develop holistic AI solutions.
Invest in Training
Provide ongoing training for employees to ensure they understand the ethical, technical, and regulatory dimensions of AI.
Adopt Advanced Tools
Leverage cutting-edge technologies for monitoring, auditing, and improving AI systems. This includes tools for bias detection, explainability, and risk assessment.
Engage Stakeholders
Maintain open communication with customers, partners, and regulators to build trust and ensure transparency in AI initiatives.
The Path Forward: AI Maturity and Competitive Advantage
As organizations in the financial and tech sectors mature in their AI capabilities, they should aim to not only manage risks but also leverage AI as a strategic differentiator. Trustworthy AI systems can enhance customer experiences, streamline operations, and unlock new revenue streams. Moreover, by adopting proactive risk management practices, these organizations can demonstrate leadership in ethical AI, setting standards for their industries.
Conclusion
Building trustworthy AI is not just a technical challenge—it is a strategic imperative for banks, financial institutions, and big tech companies. By leveraging frameworks like the NIST AI RMF and adopting best practices for risk management, these organizations can create AI systems that are reliable, ethical, and secure. In doing so, they will not only mitigate risks but also unlock the full potential of AI to drive innovation, growth, and trust in an increasingly digital world.
Interested in speaking with our consultants? Click here to get in touch
Some sections of this article were crafted using AI technology
Leave a Reply