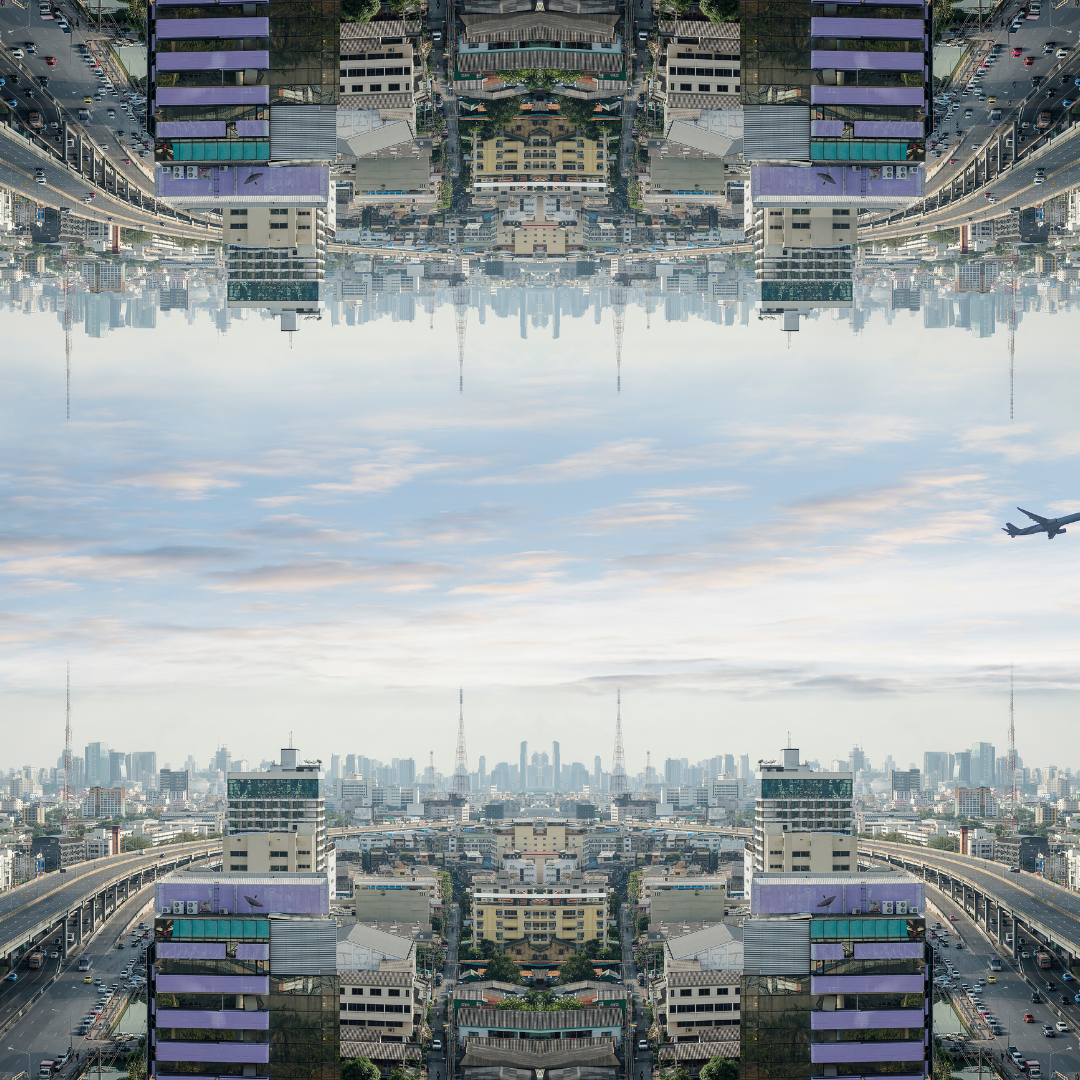
AI Risk Management: What Frameworks Should You Use?
AI Risk Management at a Glance
In today’s fast-evolving world of technology, AI risk management has quickly become a critical task in the responsible use of artificial intelligence systems. As various applications of AI continue to spread, it has become increasingly important to identify and manage risks.
AI risk management consists of identifying, assessing, and controlling possible dangers associated with the use of AI systems. This is necessary to protect data and uphold ethical standards, and to preserve the safety and reliability of deploying AI. This practice is especially valuable today where rapid progress in AI mandates proactive risk assessment to prevent undesired consequences and shape progress in AI in accordance with societal values.
By applying risk management, when AI is leveraged to its full extent by businesses and organizations, they can ensure that the fruits from AI implementations will outweigh the negative impacts, thus fostering AI innovation and trust in AI.
Managing AI Risks
With the growing adoption of AI across industries, managing AI risks is essential for organizations looking to capitalize on the potential of this disruptive technology. Despite the power of AI systems, they present unique challenges that require careful attention to prevent negative ramifications.
The most significant AI risk is the introduction of bias in decision-making processes. By learning from extensive amounts of data, AI systems can perpetuate biases and deliver unfair results if the training data is not meticulously curated. In the business context, such biases could lead to flawed hiring decisions, misguided marketing campaigns, or even discriminatory conduct, causing reputational harm and legal exposure for the organization.
Another key risk is related to safeguarding data privacy. AI technologies rely on masses of data for their operation, but mishandling confidential information can result in data breaches. When customer data is compromised as a result of an AI deployment, an organization may lose customer confidence and face financial penalties as well as regulatory sanctions.
Furthermore, the integration of AI creates new cybersecurity concerns, as cybercriminals may exploit vulnerabilities in AI algorithms. Potential consequences for businesses include service disruptions and unauthorized access to sensitive data. Consequently, businesses must employ strong security measures to shield AI solutions from cyber threats.
Operational risk is an additional aspect to consider. AI systems may malfunction or produce incorrect results, especially in the context of mission-critical processes. Such failures may disrupt business operations, causing significant downtime, inaccurate strategic choices, and reduced corporate performance.
The risk of becoming overly dependent on AI and losing critical thinking and human oversight is also a threat. While AI can drive operational efficiencies, companies must find a balance between automation and human intervention to ensure nuanced decision-making.
By understanding these AI risks, organizations can take appropriate precautions, such as conducting bias audits, establishing sound data management practices, and delivering comprehensive training to employees. By preempting these potential pitfalls, companies can exploit the benefits of AI while managing the risks, thereby securing sustainable growth and competitive advantage in the marketplace.
Exploring Leading AI Risk Management Frameworks
With the rapid development of today’s technological landscape, the use of artificial intelligence (AI) in our day-to-day lives has become more than just convenient – it has become necessary. As AI becomes more prevalent, there is a pressing demand for rigorous AI risk management frameworks to help mitigate risks and ensure the responsible, fair, and transparent operation of AI systems. Among many available frameworks, two prominent frameworks developed by the National Institute of Standards and Technology (NIST) and the International Organization for Standardization (ISO) offer structured methodologies in AI risk management.
NIST AI Risk Management Framework
The NIST AI Risk Management Framework aims to increase the trustworthiness of AI systems. A non-regulatory organization, NIST provides guidelines and standards that advocate for the ethical use of AI. A notable aspect of the NIST framework is its emphasis on transparency. The framework advocates for a methodical risk assessment of AI systems to identify, analyze, and mitigate risks. The NIST framework emphasizes a risk-based approach where professionals are encouraged to conduct ongoing assessments of AI systems for vulnerabilities and biases.
The adoption of the NIST framework boasts several advantages: the NIST framework promotes innovation by suggesting non-prescriptive guidance and bolsters public trust in AI technologies by promoting accountability and ethical AI practices. Companies can adapt the framework to adhere to their specific risk exposures, making the NIST framework a pragmatic option for organizations that deal with a range of AI applications.
ISO AI Risk Management Framework
At the global level, the ISO provides comprehensive standards in the realm of AI risk management. ISO 31000 standards serve as a universal guiding framework that enables organizations to blend AI capabilities with risk management strategies. The hallmark of this framework is its emphasis on continuous improvement and adaptability, which is essential for keeping up with the evolving nature of AI technology.
The ISO AI risk management framework advocates for a proactive approach that uncovers potential risks that arise from deploying AI. The framework incorporates strategic risk appraisal practices that span the entire life cycle of AI systems, including development, deployment, and monitoring. Companies that conform to ISO standards gain access to internationally accepted benchmarks, which not only enhance credibility but also support international cooperation and market penetration. The framework also mandates the involvement of stakeholders, ensuring that risk management protocols are inclusive and draws upon various perspectives.
Both the NIST and ISO frameworks champion the cause of trustworthy, responsible AI by offering orderly PR pathways that help companies navigate the complexities of AI risk management. By implementing these frameworks, businesses can protect against potential hazards and responsibly optimize AI capabilities. As AI solutions increasingly become integrated into industry and society landscapes, leveraging these frameworks will be critical to delivering ethical and fair AI implementations that are accountable.
Applying Risk Management Strategies
Efficient implementation of risk management strategies is key to the protection of an organization’s assets and its reputation, particularly when integrating cutting-edge technologies such as AI. Below is a structured guide to implementing risk management for AI and case studies of successful strategies adopted by leading organizations.
1. Identify Risks: The first part of applying risk management strategies is a detailed risk identification process related to AI such as data privacy, algorithm bias, or operational breakdowns. A team effort for risk assessment involving cross-functional teams ensures all-risk considerations are made.
2. Develop a Risk Management Framework: Establishing a robust risk management framework with clear protocols and procedures including risk assessment, risk mitigation, risk monitoring, and risk communication.
3. Formulate Measures Against Risks: After having identified all risks, it’s essential to develop measures to mitigate these risks. Employing data encryption, bias correction algorithms, and setting up contingency AI failure teams could be part of these measures.
4. Testing and Monitoring AI Systems: Continuous testing and monitoring are essential. Automated monitoring tools assist in tracking AI performance and adherence to risk management strategies to respond promptly to new risks.
5. Educate Stakeholders: Knowledge and awareness of the functioning and risks associated with AI among stakeholders ensure that involved parties have a clear picture of the mechanisms. Regular training sessions can increase awareness and preparedness.
Successful Case Studies:
Microsoft and Google are prominent cases of successful implementation of AI risk management. Microsoft’s “AI for Good” strategy focusing on transparency and ethical use in AI projects benefited significantly in bolstering stakeholder confidence. Google, on the other hand, has formed an AI ethics review committee tasked with identifying risks and enforcing compliance in accordance with their AI principles. These cases highlight the importance of a strategy-led risk management approach.
The application of risk management in AI not just defends against potential threats for organizations but amplifies their resilience in technology and ethics.
Ultimately, the choice of the correct framework is key to the successful management of AI risk — that framework providing a strong basis for helping organizations think through AI-related risks. Taking preemptive action will help companies to be early to identify and mitigate risks, while operating AI innovations in a safe and efficient way. By giving priority to preemptive risk management, companies can get ahead of the curve and operationalize AI strategies in line with ethical and regulatory norms. As such, a well-chosen framework enables the responsible adoption of AI, generating trust and unlocking its full potential, all while guarding against unexpected danger.
Leave a Reply